We have come a long way since the introduction of data science and machine learning. The recent study has found that the volume of business data doubles in less than 14 months. Today, the collection of data is no longer a problem, but the filtration, analysis, and maintenance of relevant information is a bigger issue.
We need to hire data science professionals, and they demand over $100k annually. Paying that sort of money for a professional is not feasible for every single organization, especially small and middle-sized companies. Google recently announced that it is going to make machine learning technology possible for every business.
The access to machine learning technology is now possible, even for small businesses due to automation. Google, Microsoft, and other companies have come up with automated machine learning tools that enable small businesses to use machine learning technology to enhance their business performance and profit.
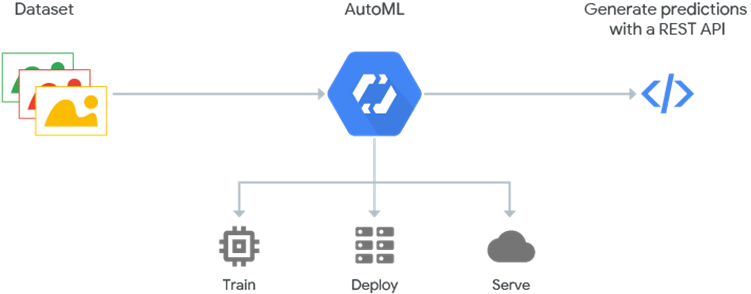
Image Source: Google Cloud
With that said, the world still needs a lot of machine learning professionals. Many machine learning professionals prefer Python for machine learning due to its features and a wide range of libraries.
According to the Gartner report, around 40% of data science tasks will be automated by 2020. The data science tools can automate some parts of data science processes, but it is not complete automation.
With that said, it has been helping a lot to accelerate the tasks. We still need data science professionals to deal with real-world problems. The algorithms are not yet able to handle messy data. The significant chunk of data science professionals often prefers performing with data science with Python for sophisticated tasks.
Automation in Data Science
Let me show you the figure right at the beginning before moving forward.
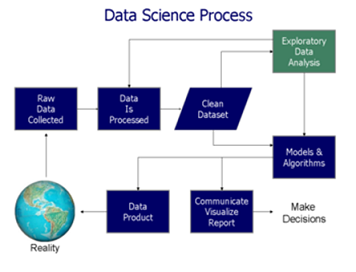
Image Source: Wikipedia
If I had to use only one word to describe the entire data science process, I would use the word “headache.” According to the recent report, the median salary of data scientists easily surpasses $100k annually. The pay will be higher in the time to come.
One needs to pay a lot of money and invest a lot of time to get insights from the collected data. The data scientists need to spend almost 50-60% of their time in data processing and the rest of their time in modeling and deployment.
The cloud platforms like Amazon Web Services, Google, Microsoft Azure, and so on make the job more comfortable, but there is still a lot of work to maintain and extract useful insights from the collected data.
The data science process has lots of inefficiencies. At first, they need to spend over 50% of their total time on processing messy real-world data. After that, there could be a need to customize models, according to specific problems.
The significant contribution of automation is making a significant portion of data processing parts automated. Secondly, the automated platforms can make tracking of various models easier from multiple parameters. The time needed to launch the algorithm is minimal.
One example of an extensive tool to handle a data science project is Alteryx. IT has come up with powerful automated solutions that can drastically reduce the data processing and model development time for smoothening the entire data science workflow. The data science platform, Alteryx, is so amazing that its share price doubled in a span of little more than a year.
Some other great tools that can help you in data science automation are Rapidminer, H20.ai, KNIME, and so on. However, the lack of skilled data scientists can create a problem despite these tools. It is where the role of automated machine learning pops in.
How is Machine Learning Transformed with the entrance of Automation?
The traditional machine learning process was too complicated. One requires to have a lot of expensive machine learning professionals working for months to come up with models to process machine learning tasks.
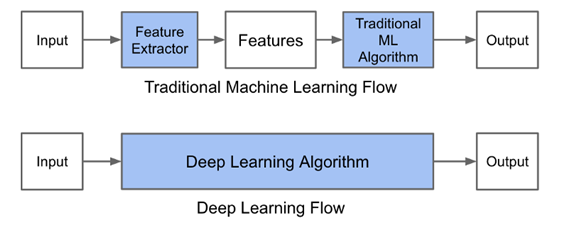
Image Source: Medium
To make traditional machine learning work, one needs to gather data, standardize data, process features, create and train the machine learning model from problems, validate the models, and deploy the models at last.
You must have heard of how machine learning is only for corporations in the past. But, that has drastically changed in recent time, and it is all due to automation. Keep in mind that the above machine learning model is a simple one. There is a lot of extra works for complicated models. Even for the simple ones, you need to spend a lot of time and money, which makes it impossible for small and medium companies.
The automation in machine learning is all about automating the entire process to make machine learning easier. The only thing you need to do is feed data to the system (not a massive volume of data). You do not need even to cross the three-figure number of images to continue with automated machine learning platforms.
Microsoft has its automl platform along with Google. Other automl platforms can do the trick for you. Using those platforms do not cost you an arm and a leg. If you check out the price, you will be surprised.
There is no need for you to create or deploy models or even test the models. The algorithm will do the job for you. It takes examples and models of historical models to process the data and use a machine learning algorithm.
Even non-statistician can implement machine learning technology with limited data, thanks to automation in machine learning. You can make use of predictive analytics and can get easy solutions for simple prediction problems without scratching your head. Numerous libraries can assist you in the automated generation of machine learning pipelines.
How are the jobs of data scientists simplified by the introduction of automation in machine learning and data science?
It is true that the introduction of automation has drastically reduced the time for completing the tasks for data scientists. They no longer have to spend their valuable time in time-consuming, monotonous works that are necessary but do not provide a lot of value.
However, the need for skilled data scientists still exist, and it will always be there in the time to come. There are challenging works for data scientists that we cannot replace with machines, such as listening to clients, figuring out the root cause of business issues, development and selection of the right solution for the specific business problem.
Just like in other types of jobs, the advancement of automation technologies will modify the tasks that data scientists need to perform. They will be able to allocate more time on things that matter rather than monotonous tasks.
Final Verdict
The automation of machine learning and data science are in the beginning stage. However, they are already making a massive impact on the business world. The huge corporations are investing in Big Data and Machine Learning technologies. We can expect a considerable improvement in these technologies shortly.
Sooner, the competitive advantage of a business will depend on how well they can use the technologies, instead of access to machine learning or Big Data technologies. I hope this article was valuable to you. If you want to add something or express your thoughts, feel free to leave a comment. I will gladly read and reply to your comment.